Enhanced force-field calibration via machine learning
Aykut Argun, Tobias Thalheim, Stefano Bo, Frank Cichos, Giovanni Volpe
Click here to see the slides.
Twitter Link: here.
The influence of microscopic force fields on the motion of Brownian particles plays a fundamental role in a broad range of fields, including soft matter, biophysics, and active matter. Often, the experimental calibration of these force fields relies on the analysis of the trajectories of these Brownian particles. However, such an analysis is not always straightforward, especially if the underlying force fields are non-conservative or time-varying, driving the system out of thermodynamic equilibrium. Here, we introduce a toolbox to calibrate microscopic force fields by analyzing the trajectories of a Brownian particle using machine learning, namely recurrent neural networks. We demonstrate that this machine-learning approach outperforms standard methods when characterizing the force fields generated by harmonic potentials if the available data are limited. More importantly, it provides a tool to calibrate force fields in situations for which there are no standard methods, such as non-conservative and time-varying force fields. In order to make this method readily available for other users, we provide a Python software package named DeepCalib, which can be easily personalized and optimized for specific applications.
Poster Session
Time: June 22nd 2020
Place: Twitter
POM Conference
Link: POM
Time: June 25th 2020
Place: Online
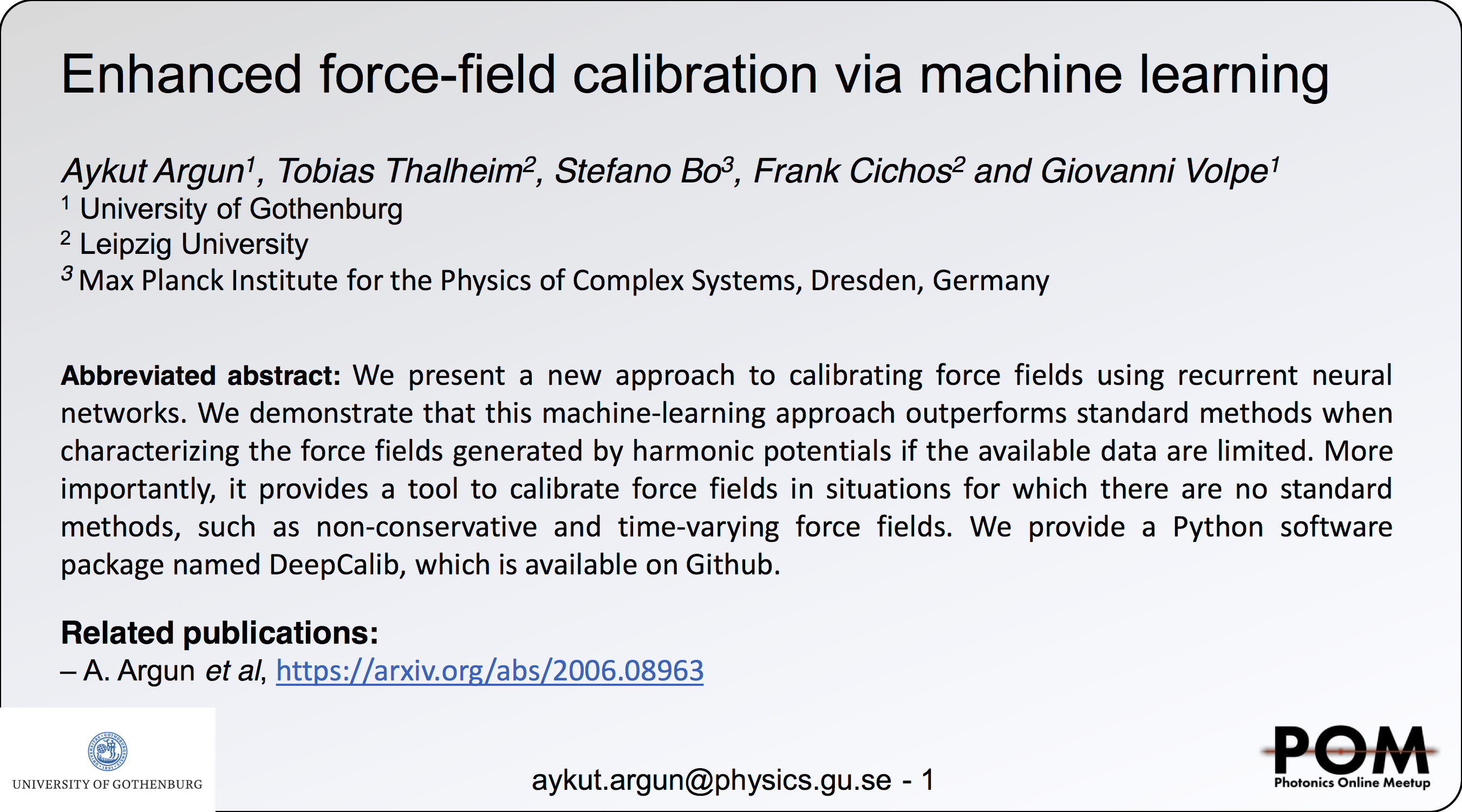
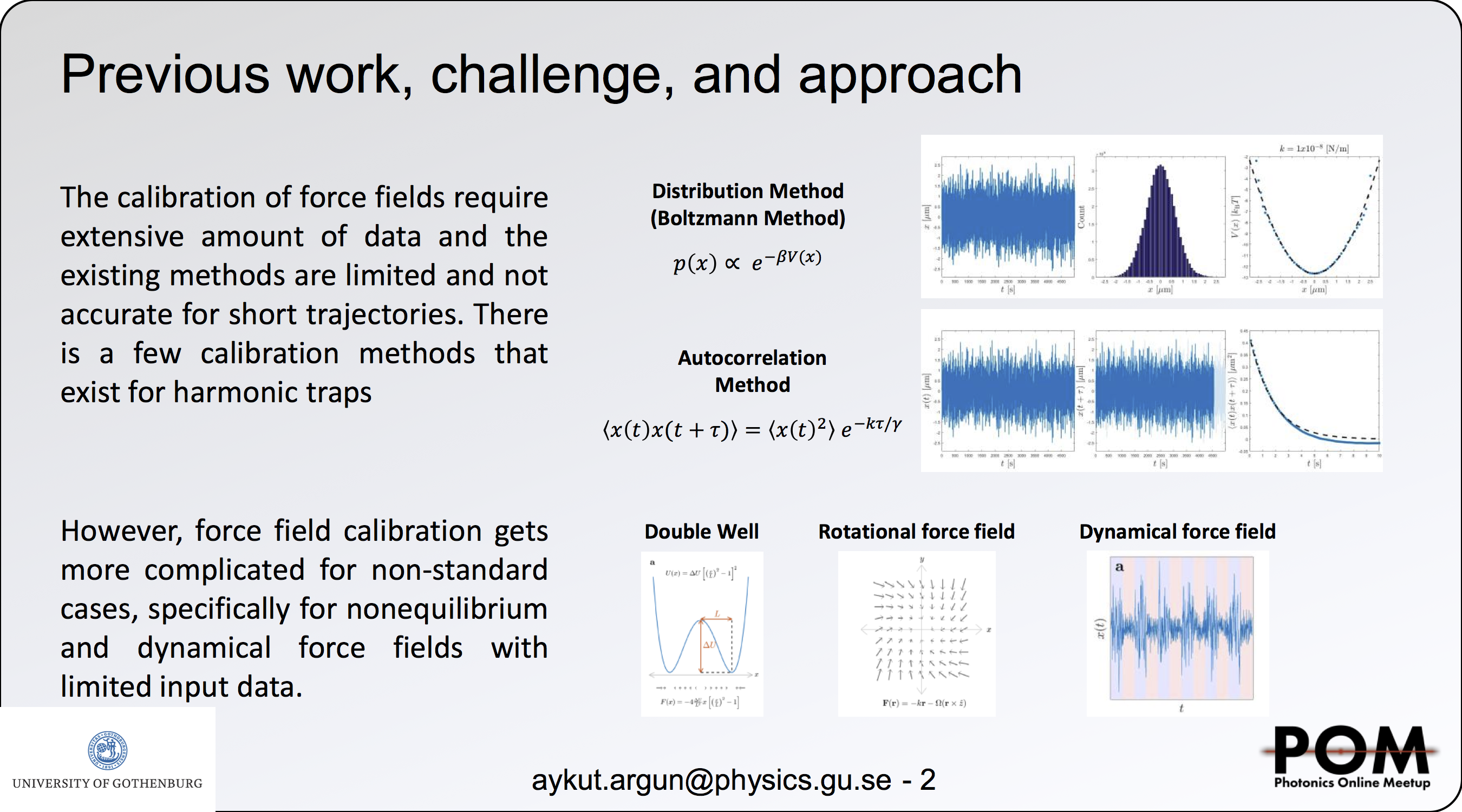
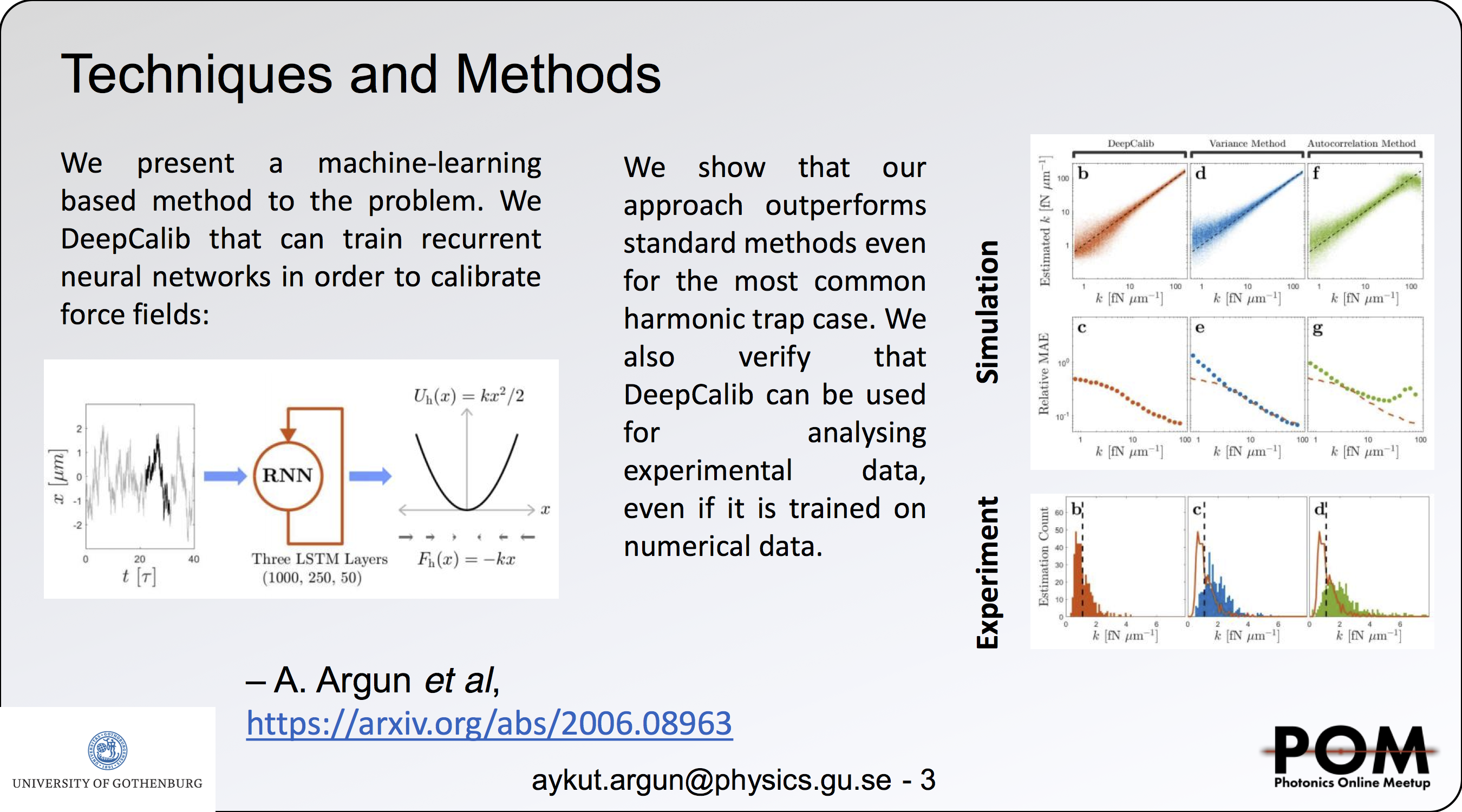
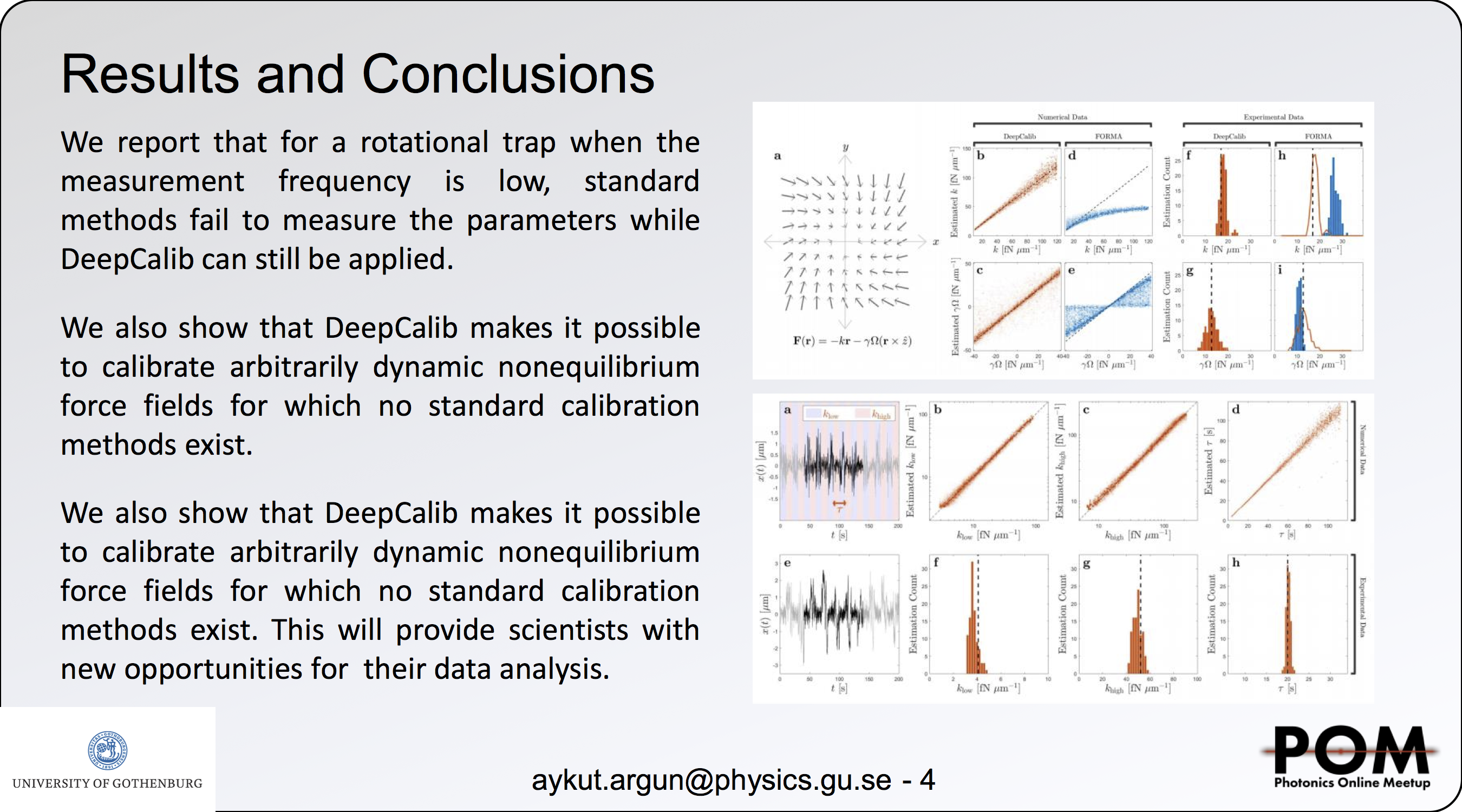